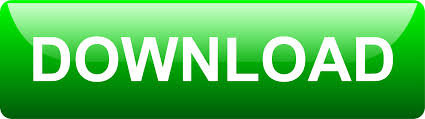

There are several uses for Principal Component Analysis, including: If the information associated with the first 2 or 3 axes represents a sufficient percentage of the total variability of the scatter plot, the observations will be able to be represented on a 2- 3-dimensional chart, thus making interpretation much easier. Principal Component Analysis can be considered as a projection method which projects observations from a p-dimensional space with p variables to a k-dimensional space (where k < p) so as to conserve the maximum amount of information (information is measured here through the total variance of the scatter plots) from the initial dimensions. Principal Component Analysis (PCA) is one of the most frequently used multivariate data analysis.

XLSTAT - Principal Component Analysis (PCA) Principles of Principal Component Analysis
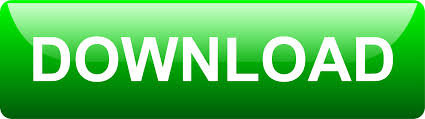